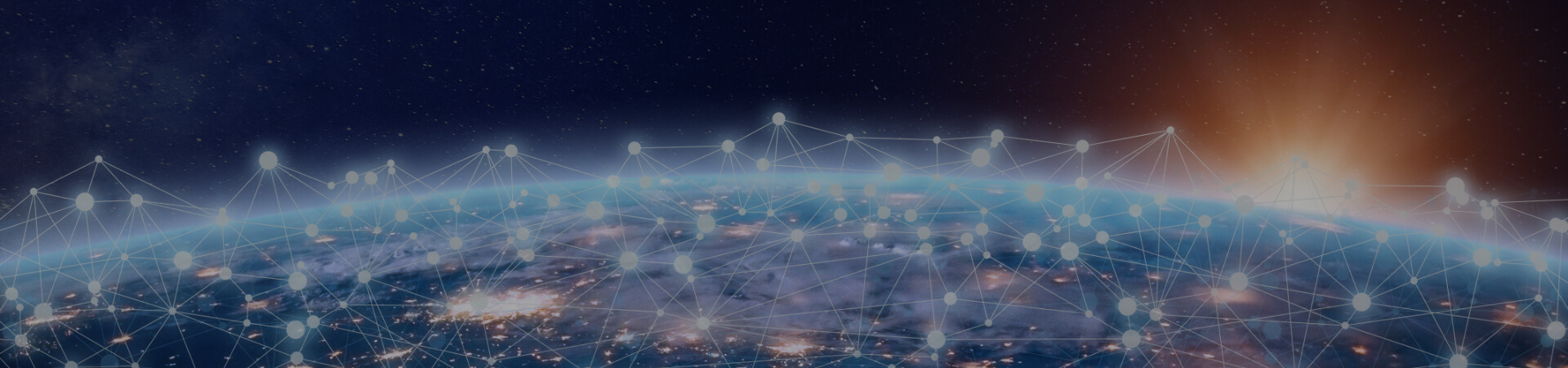
AI
Artificial Intelligence (AI) is typically defined as the ability of a machine to perform cognitive functions associated with human brains, such as perceiving, reasoning, learning, interacting with the environment, and problem solving. The concept of AI has existed since the 1950s but recent algorithmic advances, proliferation of big data, and cloud computing have propelled AI from fiction to reality. In the cloud, machine-learning algorithms process data, detect patterns in large data sets, and learn how to make predictions and recommendations. The algorithms also adapt in response to new data. As AI systems learn and adapt, they can be trained to recommend the next best action, automate repetitive tasks, and minimize human error. By 2025, the Global AI chip market is expected to reach $91 billion and will transform how consumers interact with smart devices and how smart devices interact with the world.
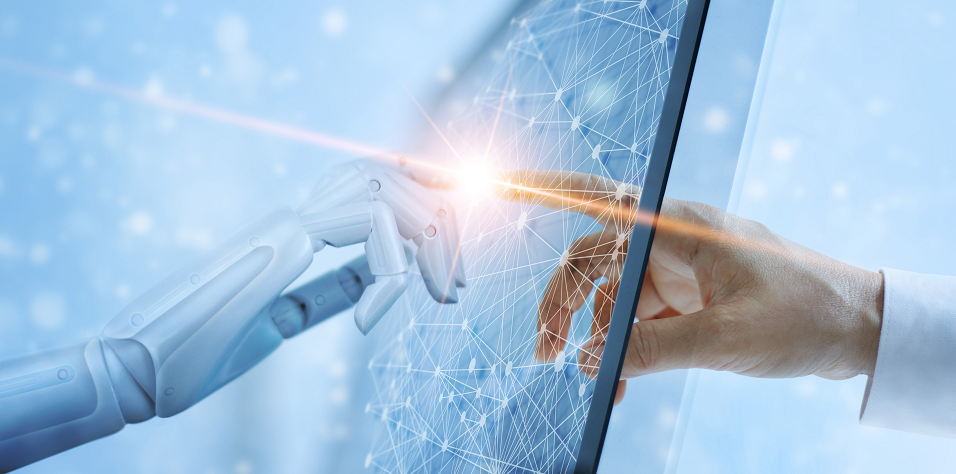
Edge Computing
While cloud computing continues to propel the growth of machine learning, the growth of connected smart devices is driving the demand for real-time data processing. The current trend is to distribute machine learning power from the cloud to smart devices at the edge and solve the privacy, latency, bandwidth, and energy consumption issues that exist today. Smart devices will be expected to carry out majority of the data analysis, sending only essential data back to the cloud.
The technologies listed below are required to develop smart devices that are capable of executing machine learning at the edge to reduce the risk of data breach, analyze data real-time, lower bandwidth cost, and reduce power consumption.
- Application-Specific Integrated Circuits (ASICs)
- FPGA/DSP/GPU/MCU...
Secure distributed intelligence
Since companies spend billions of dollars in building and training sophisticated machine learning algorithms and developing AI chips, it is critical to protect these proprietary properties and products against breaches, manipulation, and counterfeit attempts. Attackers can steal machine learning models stored in the edge devices or manipulate the input data and exploit specific vulnerabilities of learning algorithms to compromise the systems that are adaptively updated over time. Counterfeiters can also reverse engineer ASICs. Security IP can safeguard AI chips and learning algorithm by providing chip designers a random number source that can be used to create unique security keys for authentication and anti-counterfeit purposes, and secure data transmission.
Explore our solutions
Machine learning capabilities are being added to SoCs and chips to enable the deployment of edge computing in smart devices. eMemory’s security IP solutions for AI chips provide better protection for data and algorithm in-use, at-rest, and in-transit as well as enable scalable solutions that balance risks and costs.
Learn more